What is AI Scoring?
Updated: May 18, 2021
This blog is the first part of a series of entries: The Executive's guide to AI & Data Science.
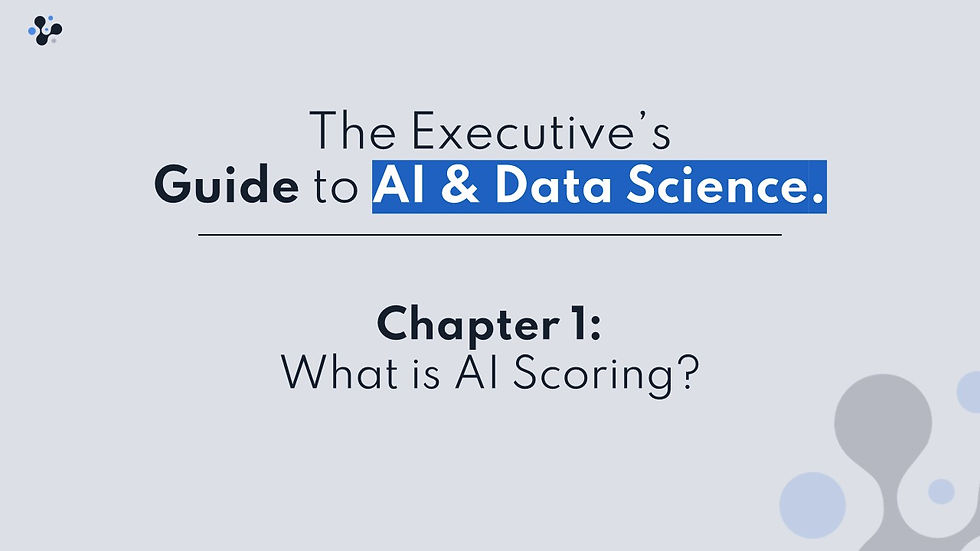
Throughout their many years of operation, banks have collected humongous amounts of data that are rarely made use of. Now with the boom of Artificial Intelligence, banks can really transform their growth leveraging their data and flexible AI platforms that enable the quick experimentation and adoption of AI.
One of the AI use cases that can have significant impact for banks is Predictive Lead Scoring as you’ll see in the below illustration. Don’t worry, we’ll explain this in a second.

Most banks now have digital applications, websites, multi-channel, and multi-touch point interaction with customers. Not to mention a wide variety of products and innovations to competitively serve customers. Each interaction with a customer leaves a data point, a trace of a customer need, an inconvenience or something to be desired.
Artificial Intelligence can learn more about these customers and automatically sort them into categories of behaviors, giving them what’s called a propensity scores. By which It scores customers on their likelihood to purchase a certain product or do a specific action. This looks like the traditional customer segmentation, but artificial intelligence can go way deeper. Rather than just basing the different classes of customers based on demographic or human preference, AI can use a lot more dimensions to accurately predict the willingness of a customer to opt in for a financial product.
Here’s an example only for demonstration purposes.
Let’s say a bank has built an artificial intelligence, and it categorized customers based on their willingness to utilize their credit cards. It gives each customer a total behavioral score based on:
● The recency of the transaction
● The location on which the transaction was made
● The data of when the customer joined the bank
● The campaign from which the customer was acquired
● The product that was purchased
● The frequency based on which the customer transacts
● The phone type the customer is using when they made the transaction
● The gender of the customer
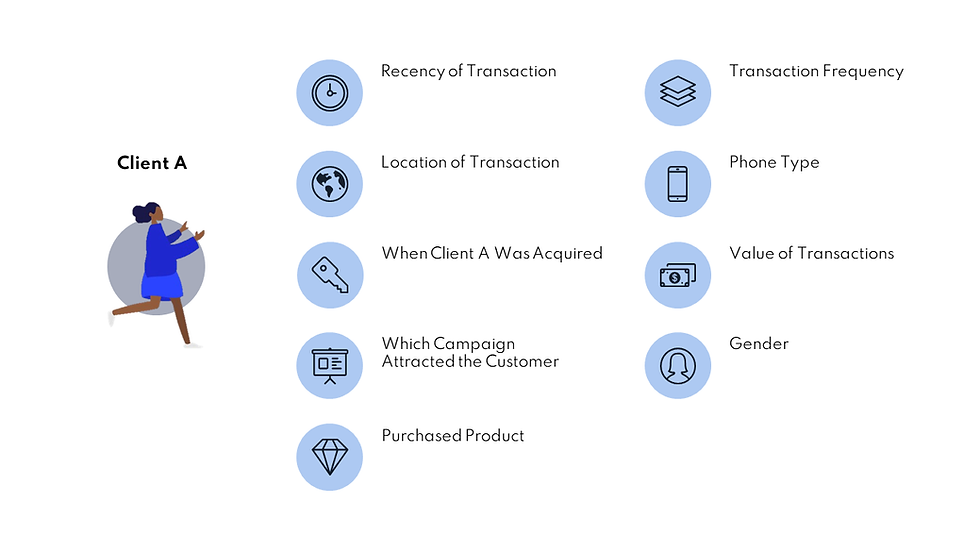
The AI is able to tell that these are the best predictors for the likelihood of a customer using their credit cards. Based on which, the AI has created 5 classes of customers:
● Class 1: The Champions [score >0.8], which are the best type of customers based on the value of their transactions
● Class 2: The Loyals [0.6-0.8], a good customer type who transacts more frequently
● Class 3: The High Potentials [0.5], are the ones who could be nurtured to be loyal or champions.
● Class 4: The At Risk [0.4], customers who are about to leave
● Class 5: The Zombies[<0.3], customers who once transacted and never came back
For each class, we can extract the best way to build a communication mix, to address them in terms of acquisitions or retention. Also, if we have a new product to launch, we can simulate whether the product would appeal to a certain class.
Moreover, business line managers can use this data to make better business decisions and benchmark performance. Also, salespeople can know how to best nurture their customer base to find cross-selling and upselling opportunities. Marketers on the other hand, can simulate campaign budget allocation based on the purpose of the initiative. Be it nurturing, retention, acquisition or a “Zombie Resurrection” campaign and choosing the best channels, and in some cases the type of content to effectively convert and excite customers.
This creates immense opportunities to sell banking products in a smarter manner and predict trends over customer needs, based on which great product mixes can be generated. Additionally, we can increase customer loyalty by recommending the products that best fit their interests and needs.
As you can imagine, this creates a very competitive growth strategy. The newly developed growth strategy puts the bank on a trajectory to create the ability to build exciting product packages This also enables the bank to focus on high value sales and marketing activities that return the highest possible ROI.
This is not as easy as it looks. It requires a lot of data science and machine learning expertise to build the models, plus highly sophisticated software engineering skills that can deploy, manage and scale the built models.
Certainly, domain expertise is critical to the success of such a project. People who manage banking products, marketers, sales and customer satisfaction leaders are all critical components of the project. They will work with data scientists to build and validate these models. In many cases, the deployed models might not have the highest accuracies. Nevertheless, they are deployed for their business impact.
Here’s a quick example of impact vs. accuracy by Synapse Analytics own Ahmed Abo El Majd:
After the models have been deployed, now comes the adoption phase, in which sales and marketing teams start incorporating AI in their daily operations. It takes a bit of time to build the human-AI relationship, but it definitely pays off in the long term.
In conclusion, AI in banking has created an opportunity to expand existing customer bases and improve customer experience. It has additionally aided in creating product package recommendations that maximize the businesses' profits as well as opportunities for targeted marketing campaigns.
Like what you read? Check out our other blogs here and stay tuned for more!
Want to know more about Synapse Analytics? Find us on LinkedIn , Facebook and Instagram or get in touch and transform your business here
Comments