Why Your Marketing Analytics Projects are not as effective as they should be?
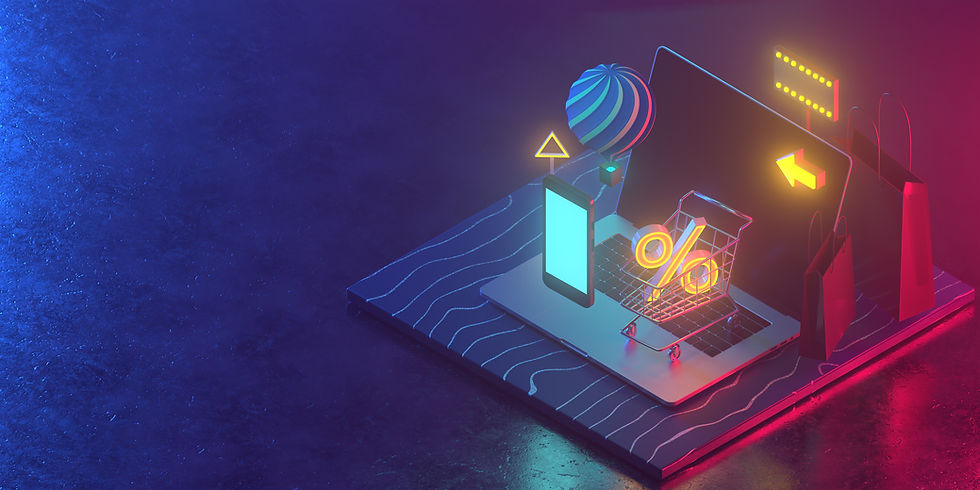
Marketing Analytics can really transform a business inside out. From creating predictable revenues, to scaling and expediting sales and growth. However, many marketing managers are not seeing this outcome in their analytics initiatives.
Often, marketing and growth managers find themselves squandered in front of some of these questions
1- We increased our spending, yet sales are still the same, why?
2- We got an unexpected surge/decline in demand, we don’t know why?
3- We’re capturing a lot of leads, however, sales are unable to close them?
4- We have the funnels, the dashboards and the reports but growth is still stagnant?
5- We have 90%+ accuracy in our deployed models however, our growth isn’t really transforming?
The root of the problem lies in the facts. Literally, the facts. Many of the analytics projects are deeply focused on the “What Happened Facts” which is useful, and getting the facts correct in the first place is a great challenge in its own right. Sometimes this can bring in value and can uplift ad performance however, it is far from being transformative, meaning seeing multiples of return on their activities.
Top Analytics teams do not only focus on the facts, they also look at what Judea Pearl and Dana Mackenzie called Counterfactuals in their amazing book The Book of Why.
Knowing and analyzing facts is an indispensable exercise. With the current dispersion of data sets, campaigns, analytics software and CRMs, the challenge of getting a vivid picture of the facts is never easy and it is not enough to change a growth curve on it's own
Working with counterfactuals is about first, knowing the facts. We combine several inputs including data, assumptions, queries, inference engines, scenario-based analysis, and regular experimentation to get different outcomes for various assumptions, as well as understanding causality and the value and cost of different actions and inactions.
To be able to execute such tasks, an analytics team must have firsthand knowledge of parametric and semi-parametric modeling, maximum likelihood analysis, propensity scores, Bayesian statistics, statistical inference, machine learning and a high marketing acumen. This is usually why analytics teams are composed of a diversified groups in terms of background and scope. (In upcoming posts, we will dive into each one and explore their impact on marketing)
It is of course challenging to hire such a team, although, having such talent will put your marketing analytics on a completely different course of growth. I believe that many analytics departments are pretty much satisfied with good marketing performance not looking at the opportunity cost of having such mindsets in their operation.
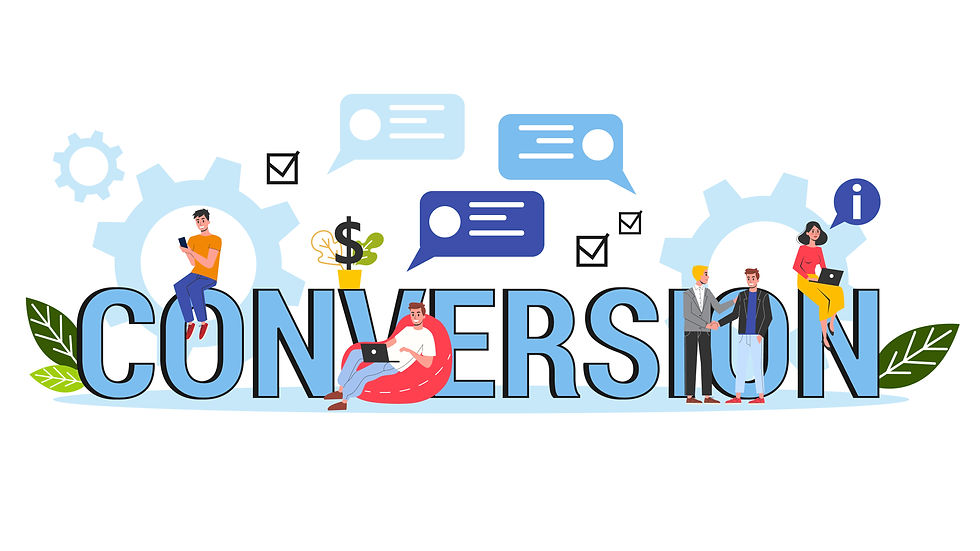
Working with counterfactuals will change the perspective from just the "whats" to the "whys" &"hows".
Here are some examples of counterfactual questions:
1- What could I have done to have converted this bounce? (Prevention)
2- Why did this customer choose product A and not B or C? (Causality)
3- Why did these 5 conversions happened? (Attributable)
4- What happens if we change A, B or C? (A/B testing)
5- Would the customer still buy product x at price ++? (What if)
In his book, Hacking Growth, Sean Ellis wrote about the Growth Equation, by which businesses would see how the different variables affect their revenues and through that, they can predict and have control over their sales. This same equation can be deducted by careful analysis of the business data, and by it, one can do a lot of comparative analysis of different scenarios.
Data is factual, and dry. You will always lack a version of the truth. Your marketing data is a finite sample from a theoretically infinite population. While most marketing analytics are focused on the ones who didn’t buy, bounced or left the cart at the last moment, analyzing and understanding the whys of a successful sale such as Why customer A bought product X, can be very fruitful to the business.
This is where the data scientists come in to extrapolate and generalize a near-accurate model of how marketing activity is affecting revenues, and with it, they can model different scenarios, build inference and causality of the "why" in success and failure towards more impactful, higher ROI decisions.
At the end, quoting the famous Greek Philosopher Democritus can be very relevant here, when he said
“I would rather discover one cause than be the king of Persia”
Check out our industry tailored solutions here
コメント