Introducing Philosophical Artificial Intelligence
Updated: Sep 15, 2022
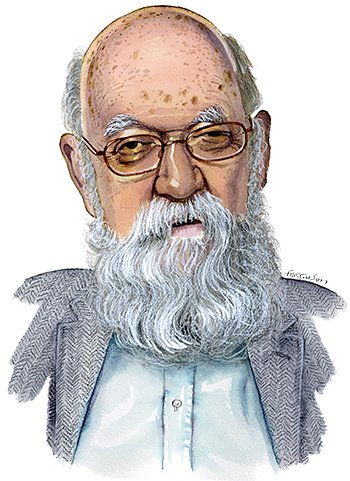
In 1979, Daniel Dennett argued for what he called ‘philosophical artificial intelligence (AI)’ rather than a ‘philosophy of AI’, maintaining that AI is not just intimately bound up to philosophy but that AI is simply philosophy. According to him, AI should be considered as “a most abstract inquiry into the possibility of intelligence or knowledge.”
I want to claim that AI is better viewed as sharing with traditional epistemology the status of being a most general, most abstract asking of the top-down question: how is knowledge possible?
– Daniel Dennett
On this view, researchers in AI and philosophy (particularly ‘epistemology’, which is the study of the nature, origin, and limits of human knowledge) ask the same question: how is human knowledge possible? Is it possible to artificially replicate and recreate it in machines?
Although the same question acted as the catalyst for all research in philosophy and AI, it took different shapes. Whereas philosophers approached the question from a Dennett-like top-down perspective, most AI researchers since the 1980s adopted a bottom-up approach. Philosophers first had to examine the human mind as is, what consciousness and cognition mean, then assess that answer’s applicability to AI’s development, or the lack thereof. The philosopher’s goal is designing and implementing abstract algorithms that can capture cognition.
On the other hand, most AI researchers’ bottom-up approach led to their attempts at identifying that which cognition can be reduced to and engineering small information-processing units in a bottom-up fashion in hopes of building or achieving high-level cognitive processes.
In that sense, AI researchers no longer engaged in the same kind of top-down abstraction:
It has seemed to some philosophers that AI cannot plausibly be so construed because it takes on an additional burden: it restricts itself to mechanistic solutions, and hence its domain is not the Kantian domain of all possible modes of intelligence, but just all possible mechanistically realizable modes of intelligence.
-Daniel Dennett
That said, the dynamic interplay between both approaches, at least to those aware of it, has produced an incredibly extensive corpus. After all, the attempt to mimic human intelligence entails (1) finding out what human cognition is made up of and (2) reproducing it in machines, in whichever order. That said, in this series, our interest resides in the discussion concerning both, simultaneously, in keeping with Philosophical AI. We will start by the ‘human mind’ or brain – you know, that clumpy thing we’re trying so hard to understand and to artificially reproduce in AI.
But first, what are we imitating – cognition, consciousness, or intelligence?
It is very easy to confuse the aforementioned terms, especially because they are convoluted in nature and are often used interchangeably. Thus, before any proper examination of the topic can begin, it is first pertinent to tidy up terminology.
Let’s start ‘simple’. Since AI is a constantly evolving field, what the term denotes is also gradually changing. Investopedia defines AI as the “simulation of human intelligence in machines that are programmed to think and act like humans”. Oxford, on the other hand, defines it as “the theory and development of computer systems able to perform tasks normally requiring human intelligence, such as visual perception, speech recognition, decision-making, and translation between languages.” Why does this seemingly minor difference matter?
If the purpose of AI is the latter –that is, being able to perform particular tasks formerly requiring human intelligence—then what should be studied is the components or minimal requirements for human intelligence. But if the purpose is to engineer machines that think and act like humans, then we are addressing total human cognition or that other ambiguous term: consciousness. This usually refers to the attempt at producing Artificial General Intelligence (AGI)–also called strong or deep AI –wherein machines are capable of understanding or learning any intellectual task that a human being can. Undoubtedly, AGI is clearly a more ambitious project than the development of AI.
What about ‘machine learning’? Is it different from either?
The latest descendent of neural-network methods, ‘machine learning’ is the study of computer algorithms that attempt to improve automatically through experience without traditional programming. That is what we use, for example, in our products Konan and AzkaVision. Although it is a subset of AI, today we find the term commonly used to denote what was previously just called AI, and sometimes interchangeably. This is because, as some argue, AI today commonly describes the attempt to implement some aspects of human abilities, such as object or speech recognition, to make machines learn some human abilities, but not the entire potential for human intelligence and cognition. In this sense, it is used to differentiate between AI and AGI. Certainly, many of those working in the field of AI will second such a view and it is admittedly a much more realistic project than the super-intelligent AI (or AGI) prophesied in the 60s.
However, many do not. A quick glance at magazine headlines, popular culture, and even peer-reviewed academic literature, will show the many grand predictions about AI today. No longer only the province of science fiction or the musings of early AI researchers, the idea that human intelligence will soon be replicated artificially, and successfully, has resurged. The serious reflection on this is credited to what is known as “The Singularity”1 the point in the future when AI will not only exceed human intelligence, but also that the machines will, immediately thereafter, make themselves rapidly smarter, reaching a superhuman level of intelligence that, “stuck as we are in the mud of our limited mentation, we can’t fathom.”
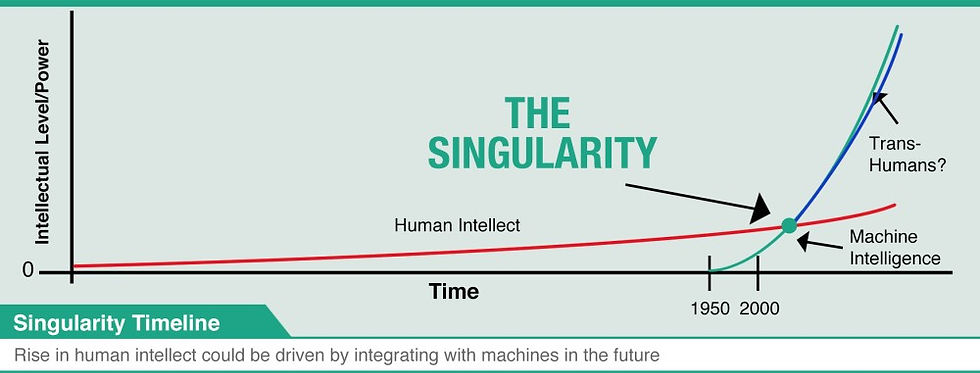
Figure 1. The Technological Singularity (innovationtorevolution, 2014)
Another question that immediately pops up is whether intelligence really refers to something different than what cognition or consciousness is.
In this article, the goal is to set the groundwork and merely pinpoint the variety of questions that will come up in this series. Now that we have identified some of them, we can move on to the more contentious issues. Needless to say, there are plenty of ways to approach this semantic minefield - which we will do over the course of this series - but for now, let’s start by taking on the most controversial of them all: consciousness!
Are you ready to rethink the notion that consciousness exists at all? Stay tuned for our next article and follow our latest news on Linkedin, Facebook and Instagram
Notes:
1. First posited by Vernor Vinge in “The Coming Technological Singularity,” (1993) in which he predicts that “within thirty years, we will have the technological means to create superhuman intelligence. Shortly after, the human era will be ended.” In the late 2000s, interest in this prediction resurged (See Eden et al., 2013)
References:
Dennett, Dennet. 1979. “Artificial Intelligence as Philosophy and as Psychology,” Philosophical Perspectives in Artificial Intelligence, M. Ringle, ed., Atlantic Highlands, NJ: Humanities Press.
Mitchell, Tom. 1997. Machine Learning. New York: McGraw Hill.
Berthold, Michael. 2019. “Artificial Intelligence Today: What’s hype and what’s real?” New Tech Forum, InfoWorld.
Comentarios