Decoding Algorithmic Bias: How AI models can foster discrimination
Two years ago, I conducted a deep dive into the intricate web of ethical concerns surrounding AI models, with a particular focus on the Middle East. Since then, the tech world has experienced a seismic shift, marked by the rapid ascent of Generative AI and Large Language Models (LLMs). In light of these transformative advancements, it is imperative to revisit the critical issues discussed in the original article and contextualize them within the dynamic landscape of today's technology.
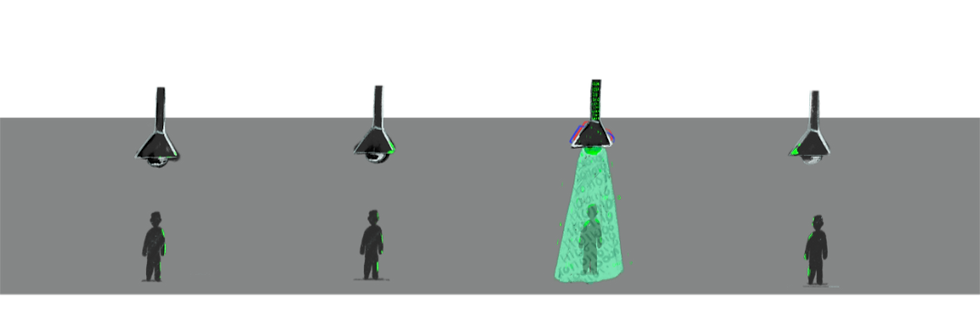
In what follows, I will explore the persisting challenges of AI biases in MENA and address how the concerns outlined in the original piece have evolved in our ever-changing technological advancements.
Generally speaking, adopting AI means better services and operations, which would directly benefit both an organization and its customers. But what happens when models fail in production? The cost of something going wrong is drastically different for both parties. For organizations, the AI tries to optimize the success metric which usually pushes the decision towards lower risks and more confident outcomes. While this makes sense from an organization’s point of view, a decision or output by a problematic model could be a life-changing - if not dangerous - event to an individual.
Here’s an overview of why (and how) an AI model can fail you, especially in MENA.
Having No Recourse
In my previous article, I discussed the lack of recourse in algorithmic decision-making by showcasing a healthcare algorithm that significantly reduced care hours without clear explanations. Tammy Dobbs, one of the affected individuals, saw a 24-hour reduction in her weekly aid hours. This reduction stemmed from a lack of transparency and accountability in the algorithm's decision-making process, leaving no effective recourse for the affected individuals. An investigation by The Verge revealed that the algorithm considered only 60 out of a long list of factors, leading to substantial care hour reductions due to minor score changes that added up.
This problem is aggravated by bureaucracy, where many of the government-collected data either had no process for correction or was tedious and slow, which means that an algorithm’s decision could take months or years to correct.
As Dannah Boyd put it, “Bureaucracy has often been used to shift or evade responsibility…. Today’s algorithmic systems are extending bureaucracy.”
Race Correction In AI Models
Today, AI models are implementing "race-corrected" features to mitigate biases and become fairer, but unfortunately, they are not without their challenges. These models sometimes rely on biased or outdated data, resulting in misdiagnoses and misallocation of resources when implemented in healthcare. An example would be the race-corrected eGFR algorithm, which is used to estimate kidney function through serum creatinine levels. Unfortunately, this algorithm reports higher eGFR values for black individuals, suggesting better kidney functions despite black individuals already facing higher rates of end-stage kidney diseases and death due to kidney failure. The rationale behind this race correction relies on claims that black individuals have higher serum creatinine concentrations due to potential muscle mass differences.
By 2020, the use of race-based eGFR values was heavily criticized, which led The National Kidney Foundation and the American Society of Nephrology to form a joint task force to look into the issue. In 2021, a new version of the eGFR algorithm was recommended for immediate implementation, and a 2023 study revealed that this revised calculation enhances kidney disease detection and reclassifies approximately 16% of lower-risk black patients, streamlining earlier access to care.
Nevertheless, race correction still exists in various other clinical algorithms such as The American Heart Association (AHA)’s Heart Failure Risk Score and Chris Moore’s STONE score. This raises questions about the necessity and impact of race correction in AI models.
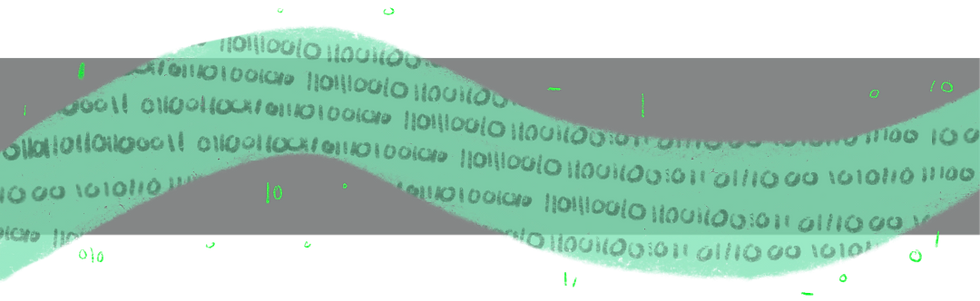
Feedback Loops – On Ground And Online
Algorithms, when not properly designed, can create feedback loops that reinforce their initial predictions. This is evident in credit scoring, where financial institutions assess customers' trustworthiness based on their spending and payment behavior using data-driven algorithms. Bad financial decisions, whether due to late payments or misuse of your financial data, can severely lower your credit score. This low score affects various aspects of life, from job prospects to loan approvals, especially impacting those with lower incomes who can't easily offset increased spending with savings. This reinforces the initial prediction.
These feedback loops are also evident in social media, where AI constructs echo chambers that continuously reinforce users' existing beliefs.
As social media permeates every part of our lives, AI can easily create echo chambers for users where they are fed viewpoints that only reinforce their own. Social media platforms achieve this through a multifaceted approach such as gathering data based on the users you follow, how you interact with the posts, and what you view on other websites to build a similarity profile where you are matched with people of similar interests and views. While this is dangerous on its own, it’s made worse with generative AI having the ability to create content that supports a certain ideology. By introducing artificially generated content that aligns with specific ideologies, these personas contribute to the self-reinforcing nature of echo chambers.
Tools built around Large Language Models (LLMs), like ThinkGPT or AutoGPT, have simplified the task of constructing fake online personas with a specific agenda, even for someone with limited coding experience. Users, whether real or generated, are exposed to content that continuously reaffirms their existing beliefs, thus perpetuating the feedback loop. This can continue perpetuating cycles of disadvantage and lead to unjust stereotyping. Consequently, social media platforms are struggling to identify and distinguish real users from bots. Platforms like Meta are currently pushing users to verify their accounts with actual paperwork. Then again, how hard is it to fake some paperwork? The authenticity of such documents remains challenging to verify, especially given the limited mechanisms available to verify their authenticity. This then poses a lot of potential risks especially when malicious intents are at play.
Individualism And Aggregation Bias
Predictive models assume that people with similar traits will do the same thing, but is this a fair assumption?
While this can accurately predict aggregate or group behavior, for an individual the equation is different: it depends solely on the person’s historical behavior. However, even this can be argued to be invalid; one example I personally love that explains this bias is by Martin Kleppmann who says:
“Much data is statistical in nature, which means that even if the probability distribution on the whole is correct, individual cases may well be wrong. For example, if the average life expectancy in your country is 80 years, that doesn’t mean you’re expected to drop dead on your 80th birthday. From the average and the probability distribution, you can’t say much about the age to which one particular person will live. Similarly, the output of a prediction system is probabilistic and may well be wrong in individual cases.”
This bias is also illustrated nicely in the context of diabetes diagnosis and monitoring. A 2022 study underscored that HbA1c levels significantly vary across ethnic groups. The adoption of a generalized and uniform HbA1c diagnostic method could lead to overdiagnosis in black people, increasing their risk of hypoglycemia, and underdiagnosis in white people.
This means that features that can promote group behavior will lead to predictions that are unjustifiably generalized and contradict the concept of human individualism.
Systemic Biases
Biases can creep into data in many forms, many of which can go unnoticed, leading to a machine learning model learning those biases. One clear source of bias is that people, companies, and societies are themselves biased; no matter the progress achieved in counteracting it, some biases always manage to slip in.
Systemic biases warrant attention as they can stem from inherently biased sources. These systemic biases can be socially detrimental. This can also lead to dignitary harms, which is the emotional distress due to bias or a decision based on incorrect data. We previously explored the evident biases in machine learning algorithms that have been trained on historical data. We’ve explored how most available datasets for human faces are biased toward white people, how the translation of non-gendered languages into gendered languages can depict stereotypes of gender roles, and how one of the infamous language models, GPT-3, learned the internet’s islamophobia and projected it on most generated text including the keyword Muslims.
This time, we’ll be exploring the biases evident from Stable Diffusion. Stable Diffusion is an open-source deep learning, latent text-to-image model released in 2022 based on diffusion techniques. One side of Stable Diffusion rapidly morphs text into fun and creative images as an outlet for personal expression, while the other side of Stable Diffusion turns the worst of biases on the internet into a bias-generative machine. This is illustrated by the following Bloomberg article headline:
“The world according to Stable Diffusion is run by White male CEOs. Women are rarely doctors, lawyers, or judges. Men with dark skin commit crimes, while women with dark skin flip burgers.”
While tools like Stable Diffusion operate effectively when properly guided, they can reveal the hidden biases they have learned if promoted with undetailed prompts. These patterns of bias become evident when assessing certain prompts. Prompts associated with high-paying jobs. like a “doctor” or a “lawyer,” generate images of people with lighter skin tones. Whereas prompts associated with lower-income positions, like a “janitor” or a “cashier,” generate images of people with darker skin tones. The models show similar biases when prompted with words like “terrorist”, “drug dealer”, or “inmate”, shadowing the pre-existing stereotypes.
Conclusion
As we navigate the ever-evolving landscape of AI and its ethical implications, it's imperative to recognize that technology's rapid advancement continues to bring both opportunities and challenges. The real-world instances highlighted in this article emphasize how necessary it is to confront the ethical dimensions of AI. The recognition of these challenges and biases is a fundamental step towards a more equitable and unbiased future. The responsible advancement of emerging technologies relies on the prioritization of transparency, fairness, accountability, and the continuous evaluation and refinement of AI models.
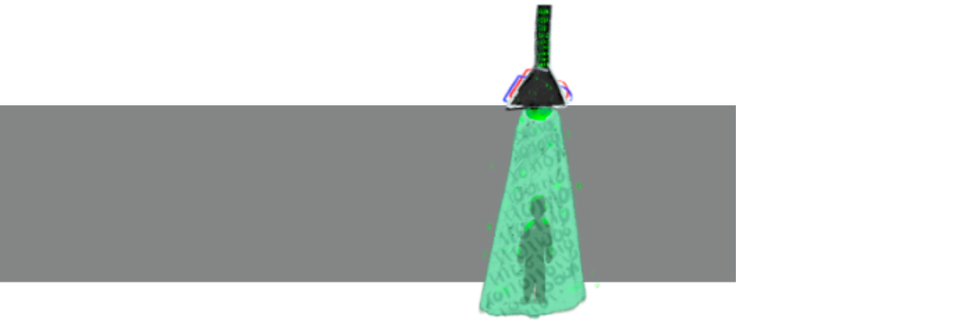
References:
Alaqeel, A., Gomez, R., & Chalew, S. A. (2022). Glucose-independent racial disparity in HbA1c is evident at onset of type 1 diabetes. Journal of Diabetes and Its Complications, 36(8), 108229. https://doi.org/10.1016/j.jdiacomp.2022.108229
Ford, C. N., Leet, R. W., Daniels, L., Rhee, M. K., Jackson, S. L., Wilson, P. W. F., Phillips, L. S., & Staimez, L. R. (2019). Racial differences in performance of HbA1c for the classification of diabetes and prediabetes among US adults of non-Hispanic black and white race. Diabetic Medicine : A Journal of the British Diabetic Association, 36(10), 1234–1242. https://doi.org/10.1111/dme.13979
Inker, L. A., Eneanya, N. D., Coresh, J., Tighiouart, H., Wang, D., Sang, Y., Crews, D. C., Doria, A., Estrella, M. M., Froissart, M., Grams, M. E., Greene, T., Grubb, A., Gudnason, V., Gutiérrez, O. M., Kalil, R., Karger, A. B., Mauer, M., Navis, G., & Nelson, R. G. (2021). New Creatinine- and Cystatin C-Based Equations to Estimate GFR without Race. The New England Journal of Medicine, 385(19), 1737–1749. https://doi.org/10.1056/NEJMoa2102953
Muiru, A. N., Madden, E., Scherzer, R., Horberg, M. A., Silverberg, M. J., Klein, M. B., Mayor, A. M., John Gill, M., Napravnik, S., Crane, H. M., Marconi, V. C., Koethe, J. R., Abraham, A. G., Althoff, K. N., Lucas, G. M., Moore, R. D., Shlipak, M. G., & Estrella, M. M. (2023). Effect of Adopting the New Race-Free 2021 Chronic Kidney Disease Epidemiology Collaboration Estimated Glomerular Filtration Rate Creatinine Equation on Racial Differences in Kidney Disease Progression Among People With Human Immunodeficiency Virus: An Observational Study. Clinical infectious diseases: an official publication of the Infectious Diseases Society of America, 76(3), 461–468. https://doi.org/10.1093/cid/ciac731
Nicoletti, L., & Bass, D. (n.d.). Humans Are Biased. Generative AI Is Even Worse. Bloomberg.com. Retrieved October 30, 2023, from https://www.bloomberg.com/graphics/2023-generative-ai-bias/#:~:text=The%20world%20according%20to%20Stable
Powe, N. R. (2022). Race and kidney function: The facts and fix amidst the fuss, fuzziness, and fiction. Med, 3(2), 93–97. https://doi.org/10.1016/j.medj.2022.01.011
Vyas, D. A., Eisenstein, L. G., & Jones, D. S. (2020). Hidden in Plain Sight — Reconsidering the Use of Race Correction in Clinical Algorithms. New England Journal of Medicine, 383(9). https://doi.org/10.1056/nejmms2004740
Comments