Can artificial intelligence help predict delirium?
Introduction
It is inevitable: healthcare will be transformed by artificial intelligence (AI). Over the past decade, AI has been increasingly used - in combination with human judgement - across a wide range of medical fields. The MENA region should not be a stranger to this phenomenon as our healthcare systems could benefit immensely from the incorporation of AI. This article will provide an overview of the state of AI in healthcare with a specific focus on its use in identifying and diagnosing delirium in patients. It will highlight examples of the technology’s applicability in the field of psychiatry its potential for growth in the MENA region.
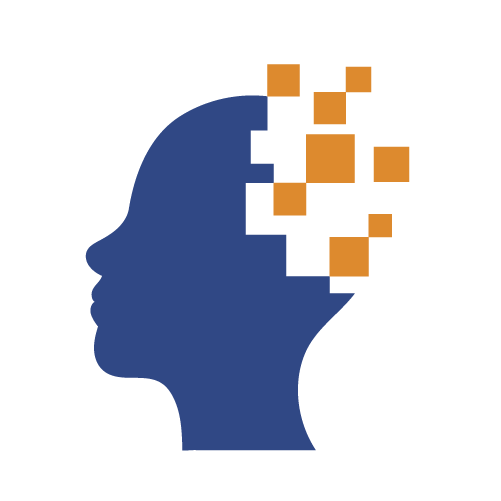
Given the increasing availability of patient data in the region, as well as the enormous advances in AI and high-performance computing, AI-enabled technology can completely transform our healthcare systems. They can be used to facilitate disease detection and prediction and can also identify appropriate treatments. There are many promising examples showcasing the varying applications of AI in healthcare:
PathAI, an AI-powered pathology technology provider aims to integrate human and AI resources to improve the diagnosis of diseases such as breast cancer. Other companies have employed AI for treatment, rather than diagnostic enhancement. For example, BioXcel Therapeutics combines existing licensed pharmaceuticals and/or clinically verified product candidates with big data and machine learning algorithms to uncover novel therapeutic indicators. The company’s first medication was authorized for use in 2022 as the first of its kind.
Use of AI in psychiatry
The applicability of AI is not just limited to physiological diseases. AI is also used to diagnose, monitor, and treat a wide array of psychiatric diseases, as well as to enhance the efficiency of the clinicians administering the treatment.
In order to observe and detect psychiatric diseases, researchers depend on a number of techniques, including electronic health records (EHR). AI can play an integral role in utilizing this data to help predict and diagnose diseases with a high accuracy rate.
For example, speech is recognized as one of the key identifiers used by psychiatrists to diagnose certain mental disorders such as psychosis. In 2018, a group of researchers were able to utilize automated machine-learning speech classifiers to help predict psychosis in patients (Corcoran et al. 2018). The system – which had an 83% accuracy rate of predicting the onset of psychosis - accepted speech transcripts as input, performed speech preprocessing, latent semantic analysis to identify word associations, and part-of-speech tagging analyses to tag each word according to its grammatical function.
If we can use AI to analyze massive datasets such as EHRs, we may be able to develop algorithms that can predict anything from who is likely to self-harm, to who is likely to develop a certain mental disorder. This begs the question: what about the diseases that humans themselves often misdiagnose? Is it possible to create AI models that can identify health risks that often go unnoticed?
An Overlooked Health Danger: Delirium
Delirium is an acute state of brain failure characterized by unexpected disorientation, a fluctuating course, inattention, and frequently an abnormal degree of awareness. Although delirium is a common illness, it is often overlooked in hospitals. Approximately 55% to 80% of delirium cases in the United States are undetected by healthcare teams (Mattison, 2020). So just how common is it, and why is it often undetected?

Research has found that in intensive care units (ICUs), prevalence rates range from 47.1% to 84.2%. In a study of delirium prevalence across 35 hospital departments, 84.2% of delirium cases were misdiagnosed. The difficulty of detecting delirium is due, in part, to the variability of factors that offset delirium in a patient, including surgery, physical restraints, severe illness such as sepsis and stroke, and many others. While there are several screening methods for delirium, health practitioners must receive specialized training in these techniques to correctly and quickly identify the disease (Mattison, 2020).
Delirium’s prevalence in the MENA region
Delirium’s prevalence and frequent misdiagnosis is just as much of a problem in our part of the world. In 2022, a study was undertaken in several ICUs and tertiary hospitals in Saudi Arabia to determine the daily prevalence of delirium. The findings indicate that 45.9% of patients were diagnosed with delirium (Alqadheeb et al., 2022). Similarly, in a study conducted in 2021 on 588 Egyptian patients, 33.7% of the participants were diagnosed with delirium (Ibrahim et al., 2022).
Despite its prevalence, delirium is often undetected by clinicians due to the lack of specialized training. A 2021 study at ICUs affiliated with Menoufia University Hospital in Egypt to assess nurses' knowledge of delirium revealed that most nurses needed a comprehensive description of delirium to recognize it. The majority of investigated nurses never assessed ICU patients for delirium, which was hypothesized to be related to the absence of delirium testing and assessment (Hassnin Eita et al., 2021).
Similarly, in a study carried out in Palestine in 2018, most healthcare practitioners reported not having completed delirium assessments previously. The authors of the study discuss the importance of hospitals using recognized instruments for measuring delirium in ICUs and teaching physicians and nurses how to recognize and treat delirium early on (Salameh et al., 2021). Recent research confirms this view and indicates that a significant majority of hospital physicians and nurses in the MENA region lack adequate training in delirium diagnostic methods.
Consequences of Delirium
Left undiagnosed, delirium can have devastating consequences. Patients with delirium are considerably more likely to die within six months than those without delirium (Maldonado). In addition, the length of time they need to be in the hospital is 5 to 10 days longer, and they are more likely to require long-term care (Kuehn, 2010). The consequences often also extend beyond the patient alone: care for patients with delirium costs at least twice as much as care for equally sick patients without delirium (Kuehn, 2010). These individuals may also present doctors and family members with emotional and physical issues. Patients with hyperactive delirium, for instance, may attempt to get out of bed or remove medical equipment, whereas those with hypoactive delirium may express a desire to die.
The case for AI models in delirium detection
On a global scale, current delirium evaluation techniques have several significant flaws. Firstly, the questionnaire-based nature of these technologies makes their incorporation into standard clinical workflow unfeasible. Manually examining all patients’ characteristics to determine whether they may develop delirium is a difficult and complex undertaking. Symptoms of delirium tend to coincide with other neuropsychiatric diseases’ symptoms, such as depression, making a clear diagnosis less straightforward.
In addition, current evaluation techniques require the patient to exhibit clear indications of delirium that often present themselves in the later stages of the disease or require the patient to have obvious risk indicators upon arrival to the hospital, making early detection and prevention a difficult task (Boettger et al., 2021). This is where AI can help.
EHRs and machine learning have made it possible to create models that can predict delirium for different types of hospitalized patients receiving different care. Prediction models can generate a risk score for every patient to analyze their condition and their likelihood to suffer from delirium, allowing physicians to prioritize patients with a greater propensity to developing delirium and respond swiftly.
Using a number of indicators based on characteristics of ICU-admitted patients, several models have been developed to aid early detection and diagnosis. Some models estimate the risk score for delirium based on the post-operative status of patients (Racine et al., 2021), where others predict delirium in individuals admitted to hospital rooms (Jauk et al., 2022) as opposed to ICUs (Bishara et al., 2022).
Successful Deployments of Delirium Prediction AI-based Model
A group of Austrian researchers built a machine learning model for the prediction of delirium risk and examined its effectiveness in a clinical setting over seven months. The clinical context in which the model was assessed was distinct from the hospital where the model's training, validation and test data were collected, proving its generalizability. The model was trained using data acquired from EHRs in KAGes in Austria, and later retrained using data extracted from hospital EHRs (Jauk et al., 2022).
The results were promising. Physicians assessed the risk of delirium for 93 patients admitted to the trauma surgery department. Standard assessment tools were used within 48 hours of admission. The machine learning risk prediction model developed by the researchers predicted the risk of delirium at several intervals: the moment of admission, the evening of admission, and the evening of the second day of admission. The senior physician on call determined that 29 of the 93 patients had a high risk of delirium. All 29 of these patients were predicted by the delirium prediction model to be at high risk or very high risk, with a sensitivity[1] of 100 percent.
Ultimately, the model was able to predict which patients developed delirium with a 90.6% accuracy rate. In addition, the model gave interpretations of its resultant risk scores via a visualization that provided a ranked list of features by their relevance for the prediction and patient-specific values for all feature groups that contributed to the output risk score (Jauk et al., 2022).
Pathways for implementation in the MENA region
There is immense potential for the application of this type of technology in the MENA context. However, standards must be met for the successful implementation of this AI-enabled solution in the region’s healthcare systems. The likelihood of achieving similar results to the Austrian context rests on whether these standards are followed.
The implementation of this AI-based prediction model depends on the availability of quality data. This is where the importance of EHRs comes in – in order to successfully adopt this tech-enabled model, EHRs need to serve as the foundation of our healthcare systems.
At present, EHRs are being deployed in a network of hospitals across Egypt. Recent research on COVID patients conducted in 2020 utilized data extracted from EHRs at four public hospitals and one national institute, indicating the availability of robust data from EHRs (AbdelGhaffar et al., 2022). Another recent study undertaken to evaluate the quality of EHRs obtained data at random from seven different primary health center (PHC) units in Alexandria, highlighting the widespread adoption of EHRs in Egypt. At the time of the study, 45 out of 75 PHC units in Alexandria had operational electronic records (Noureldin et al., 2022), all of which are owned by the Ministry of Health and Population.
However, research has revealed a number of obstacles Egypt faces in implementing wide-spread, comprehensive EHRs. Noureldin et al. (2022) assessed the completeness and correctness of documentation in electronic medical records in Alexandria by comparing paper medical records to their electronic counterparts. Compared to paper-based records, e-records were found to contain much less full data, with varying levels of completeness. The researchers predict that this incompleteness is due in part to physicians' assumption that the data in these e-records is intended to act as a subset of the data in paper records, not an exact replica (Noureldin et al. 2022).

This disconnect was attributed to the concurrent use of both paper and digital records. As such, researchers propose that the dual usage of records should be temporary and limited to the e-records deployment period. After which, paper records should be entirely replaced by e-records (El Hadi, 2022). In addition, physicians should be educated and trained on the benefits and application of such technologies. Correct usage of EHRs is key, as the success of machine learning models depends on the quality of the input data.
Further, in order to properly implement AI into our healthcare systems, it is also crucial to examine the scalability of implementation. Given that not every hospital will have access to the technical infrastructure, sufficient data, and relevant know-how, clinical institutions and commercial organizations can gain an advantage by clustering their resources; digital health, AI, human resources, and biomedical research. A collaboration between commercial institutes and public hospitals with the goal of exchanging digital resources and large-scale data could be an example of such clustering.
Explainability and trust are also key factors that determine the successful implementation of these technologies in the region. Patients and professionals should receive clear clarifications regarding the intended use of AI in the healthcare system. It is essential to specify if the AI-enabled models are being utilized to provide a definitive prognosis, or to simply act as a decision-making aid (ElHadi, 2022). Patients and clinicians have the right to understand how an AI model arrived at its prediction. Therefore, AI models utilized in healthcare must offer feedback and be explainable.
Finally, funding is crucial to replicating AI's success in the MENA region. Dedicating a portion of our digitization efforts and funding capabilities towards incorporating AI in healthcare is vital. In addition to consolidating funds to support scaling AI development, it is necessary to incentivize local and private health institutions to collaborate and work together towards the same research and development objective.
Notes:
[1] Sensitivity is a measurement of the proportion of delirious patients whose condition was accurately anticipated.
References:
Corcoran, C. M., Carrillo, F., Fernández‐Slezak, D., Bedi, G., Klim, C., Javitt, D. C., ... & Cecchi, G. A. (2018). Prediction of psychosis across protocols and risk cohorts using automated language analysis. World Psychiatry, 17(1), 67-75.
Mattison, M. L. (2020). Delirium. Annals of internal medicine, 173(7), ITC49-ITC64.
Boettger, S., Zipser, C. M., Bode, L., Spiller, T., Deuel, J., Osterhoff, G., ... & von Känel, R. (2021). The prevalence rates and adversities of delirium: Too common and disadvantageous. Palliative & supportive care, 19(2), 161-169.
Alqadheeb, N. S., Hashhoush, M. S., Alharthy, A. M., Mahmood, N. N., Alfardan, Z. A., Amin, R., ... & Almuslim, O. M. (2022). Point prevalence of delirium among critically ill patients in Saudi Arabia: A multicenter observational study. International Journal of Critical Illness and Injury Science, 12(2), 70.
Ibrahim, M. H. E. D., Elmasry, M., Nagy, F., & Abdelghani, A. (2021). Prevalence and risk factors of delirium and subsyndromal delirium in older adults. The Egyptian Journal of Internal Medicine, 33(1), 1-6.
Hassnin Eita, L., & Mohamed Abdelaal, H. (2021). Nursing Knowledge and Practices Regarding Delirium as an Under-Recognized Syndrome in Intensive Care Units (ICU psychosis). Egyptian Journal of Health Care, 12(1), 904-920.
Salameh, B., Al Razeeni, D. M., Mansor, K., Abdallah, J. M., Ayed, A., & Salem, H. (2021). Delirium in Intensive Care Units: Perceptions of Physicians and Nurses. Critical Care Nursing Quarterly, 44(4), 393-402.
Kuehn, B. M. (2010). Delirium often not recognized or treated despite serious long-term consequences. JAMA, 304(4), 389-395.
Wong, A., Young, A. T., Liang, A. S., Gonzales, R., Douglas, V. C., & Hadley, D. (2018). Development and validation of an electronic health record–based machine learning model to estimate delirium risk in newly hospitalized patients without known cognitive impairment. JAMA network open, 1(4), e181018-e181018.
Jung, J. W., Hwang, S., Ko, S., Jo, C., Park, H. Y., Han, H. S., ... & Ro, D. H. (2022). A machine-learning model to predict postoperative delirium following knee arthroplasty using electronic health records. BMC psychiatry, 22(1), 1-11.
Racine, A. M., Tommet, D., D’Aquila, M. L., Fong, T. G., Gou, Y., Tabloski, P. A., ... & Jones, R. N. (2021). Machine learning to develop and internally validate a predictive model for post-operative delirium in a prospective, observational clinical cohort study of older surgical patients. Journal of general internal medicine, 36(2), 265-273.
Bishara, A., Chiu, C., Whitlock, E. L., Douglas, V. C., Lee, S., Butte, A. J., ... & Donovan, A. L. (2022). Postoperative delirium prediction using machine learning models and preoperative electronic health record data. BMC anesthesiology, 22(1), 1-12.
Jauk, S., Veeranki, S. P. K., Kramer, D., Högler, S., Mühlecker, D., Eberhartl, E., ... & Leodolter, W. (2022). External Validation of a Machine Learning Based Delirium Prediction Software in Clinical Routine. In dHealth 2022 (pp. 93-100). IOS Press
AbdelGhaffar, M. M., Omran, D., Elgebaly, A., Bahbah, E. I., Afify, S., AlSoda, M., ... & Shousha, H. I. (2022). Prediction of mortality in hospitalized Egyptian patients with Coronavirus disease-2019: A multicenter retrospective study. Plos one, 17(1), e0262348.
Noureldin, M., Mosollom, R., & Hassan, S. Z. (2014). Quality of documentation of electronic medical information systems at primary health care units in Alexandria, Egypt. EMHJ-Eastern Mediterranean Health Journal. 2014; 20 ( 2): 105-111.
El Hadi, M. (2022). Digital Healthcare Supported by Artificial Intelligence with Emphasis on Egypt. مجلة الجمعية المصرية لنظم المعلومات وتکنولوجيا الحاسبات, 27(27), 5-17.
Comments